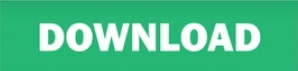
The company also does provide Data APIs to Enterprise customers.
#Amazon redshift alternative software
This is a SaaS software helping real estate professionals keeping up with their prospects and leads in a given neighborhood/territory, finding out (thanks to predictive analytics) who's the most likely to list/sell their home, and running cross-channel marketing automation against them: direct mail, online ads, email. #Devops - GitHub, Travis CI, Terraform, Docker, Serverless See moreīack in 2014, I was given an opportunity to re-architect SmartZip Analytics platform, and flagship product: SmartTargeting. To build #Webapps we decided to use Angular 2 with RxJS #Data - Amazon RDS, Amazon DynamoDB, Amazon S3, MongoDB Atlas #Eventsourcingframework - Amazon Kinesis, Amazon Kinesis Firehose, Amazon SNS, Amazon SQS, AWS Lambdaģ. #Microservices - Java with Spring Boot, Node.js with ExpressJS and Python with FlaskĢ. To build our #Backend capabilities we decided to use the following:ġ. The event-driven architecture is made up of highly decoupled, single-purpose event processing components that asynchronously receive and process events. We also decided to use Event Driven Architecture pattern which is a popular distributed asynchronous architecture pattern used to produce highly scalable applications. Microservices modularity facilitates independent updates/deployments, and helps to avoid single point of failure, which can help prevent large-scale outages. You can gain modularity, extensive parallelism and cost-effective scaling by deploying services across many distributed servers. Microservice architecture style is an approach to developing an application as a suite of small independently deployable services built around specific business capabilities. We decided to go with Microservices architecture as we wanted scale. We are in the process of building a modern content platform to deliver our content through various channels. While this approach had a couple of bumps on the road, like re-triggering functions asynchronously to keep up with the stream and proper batch sizes, we finally managed to get it running in a reliable way and are very happy with this solution today. We went ahead and implemented the Lambda-based approach in such a way that Lambda functions would automatically be triggered for incoming records, pre-aggregate events, and write them back to SQS, from which we then read them, and persist the events to BigQuery. In the past we had workers running that continuously read from the stream and would validate and post-process the data and then enqueue them for other workers to write them to BigQuery. Before ingesting their data into the pipeline, our mobile clients are aggregating events internally and, once a certain threshold is reached or the app is going to the background, sending the events as a JSON blob into the stream. Once events are stored in BigQuery (which usually only takes a second from the time the client sends the data until it’s available), we can use almost-standard-SQL to simply query for data while Google makes sure that, even with terabytes of data being scanned, query times stay in the range of seconds rather than hours. While this does sound complicated, it’s as easy as clients sending JSON blobs of events to Amazon Kinesis from where we use AWS Lambda & Amazon SQS to batch and process incoming events and then ingest them into Google BigQuery. In order to accurately measure & track user behaviour on our platform we moved over quickly from the initial solution using Google Analytics to a custom-built one due to resource & pricing concerns we had.
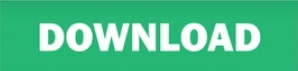